Artificial intelligence as toolset for analysis of public opinion and social interaction in marketing: identification of micro and nano influencers
- 1Management Department, SBS-Swiss Business School, Zurich, Switzerland
- 2Department of Sociology, College of Humanities and Sciences, Ajman University, Ajman, United Arab Emirates
- 3Humanities and Social Sciences Research Center (HSSRC), Ajman University, Ajman, United Arab Emirates
- 4Department of General Subjects, Humanitarian and Technical Academy, Kokshetau, Kazakhstan
In the conditions of digitalization, which has penetrated all spheres of human life and society, artificial intelligence (AI) and ultra-precise neural networks influence our thinking and form consumer demand and loyalty. Modern AI applications cover all the essential areas of activity: education, manufacturing, marketing, management, law, medicine, and e-commerce. The study aims to build a methodology for analyzing the influence of public opinion in social networks, particularly micro and nano influencers, through AI and machine learning on the effectiveness of companies' marketing activities. The authors studied the stages of consumer interaction with marketing profits and highlighted the role of AI technologies in each of them. They showed that public opinion was the main driving force for effective sales of products on the Internet. Based on empirical data, a strong direct relationship was found between the number of influencers and the dynamics of marketing profit: it was revealed that the correlation coefficients between the marketing profit index and the number of micro and nano influencers were 0.86–0.99 in all surveyed companies implementing AI technologies, regardless of the market niche. Based on the expert method, the company's marketing system is investigated. An integral indicator of its effectiveness is introduced, consisting of sub-indices of analysis, diffusion and marketing information management. The authors considered the factors of profit from marketing activities of five companies actively implementing AI in brand promotion, including those using the leadership opinions of influencers. The applied value of the developed methodology is the possibility of using it to construct Internet marketing strategies by companies. By permanently monitoring the relationship between the marketing profit index and the degree of brand popularization in social networks, companies can manage sales at all stages of consumer communications, optimizing the costs of influencers promoting products on different social platforms. The prospects for further research are to study the relationship between the degree of interactivity of the influencer with the audience, the content posted by it with the conversion rate and sales volumes; grouping and identification of influencers based on the clustering method.
1. Introduction
Artificial intelligence reveals to modern companies unprecedented horizons for the growth of the efficiency of all business processes and capitalization, while simultaneously setting new standards and challenges for management. Today, a unique event is becoming not only and not so much the production of goods, but its competent and effective promotion and sale. The analysis of social interaction and public opinion through artificial intelligence is at the heart of the profitable marketing activities of modern progressive brands. The COVID-19 pandemic had a significant impact on customer behavior, which can be traced to offering anxiety-mitigating and therapeutic benefits, as well as increasing the speed of individual customers' habit formation (Gerlich, 2021).
Modern research confirms that it is not the presence of AI technologies as such in the marketing arsenal of companies, but the effective management of new competitive advantages that they are able to produce that expands the commercial prospects of brands. The market success of the developed innovations and the management of sales processes depend on the effectiveness of AI implementation and continuous improvement of the quality of opinion mining and their semantic recognition and analysis (Jarek and Mazurek, 2019; Li et al., 2020; Jang et al., 2022).
Social interaction based on social capital, trust in leadership opinions is a fertile basis for increasing consumer demand and sales, and effective intellectual analysis of big data of public opinion is a tool for demand management. World practice shows that the popularity of the tool of intellectual analysis of public opinions in marketing is growing daily. That can be seen both in the growth of investments in leaders of public opinion about brands (up to $ 3.69 billion in 2021 and a forecast of up to $5 billion in 2023) and in the number of marketers working with influencers (more than 60% of marketers in 2021 compared to 55.4% in 2019) (Retailers, 2022).
Microblogging is developing as part of the Web 2.0 concept, in which each of the web users becomes a producer of their own content. This content can have independent value and act as a tool for almost free marketing. The more users participate in interactive social interaction with a blogger, the more influence he has on public opinion. This influence can be monetized through advertising, political discourse, or otherwise (Currier, 2019; Du, 2020).
The relevance of AI and machine learning technologies research lies in finding new methods for analyzing the influence of influencers' opinions on consumer demand, sales and marketing profit. Based on automated search and analysis of the information necessary for the company, marketers build market strategies and plan and control the dynamics of consumer demand. In turn, IT specialists and web analysts extract strategic sensory information from speech, text and images, systematize it, recognize and evaluate emotions, create encryption protocols, and implement methods of intelligent analysis of crucial market indicators: prices, supply and demand—increase the level of development of the bank of models and methods of data analysis such as arithmetic, statistically, predictive operations, graphical processing, etc.
Thanks to the symbiotic marketing efforts of marketers, IT employees, and web analysts, companies repeatedly increase sales of products that are not innovative or unique. Through artificial intelligence, it is now possible to study the complete profile of a client as a user of the Internet and social networks, a generator and a consumer of web content. This information will enable marketers to develop products down to the smallest detail and implement effective marketing strategies, expanding their market presence and increasing marketing profits and gross profit.
AI helps brands and consumers learn more about each other personalize contacts as much as possible and establish an effective communication system. Modern machines skilfully process, read and understand human speech and images interpret the environment, process natural language and synthesize oral and written speech, generating tens of thousands of lines per second. The article's purpose is to deepen the analysis of the influence of public opinion through artificial intelligence on marketing business processes and their productivity.
The practical significance of the paper and its contribution to existing world research lies in the applied value of the developed methodology for analyzing the impact of the activities of public opinion leaders on the effectiveness of marketing companies. Based on the study of the relationship between the number of influencers and the marketing profit index, companies expand the tools of product sales management and reduce or increase the costs of opinion leaders in time according to the stages of consumer interaction.
2. Literature review
2.1. Artificial intelligence
Artificial intelligence permeates all cells of the value chain, starting with the development of innovations and the production of consumer value and ending with the organization and management of marketing (Jarek and Mazurek, 2019). Management tasks and functions related to strategic planning, marketing and customer support have risen to a qualitatively new level thanks to AI technologies' emergence and permanent modernization (Gil et al., 2020).
At the same time, the critical issue of artificial intelligence research is machine learning as a technology for extracting helpful business information from an immense data set. In this regard, the authors study the structure of machine learning and deep learning as its methods, including machine education, inductive learning, learning by analogy, explicable learning, learning based on neural networks, and knowledge discovery (Dargan et al., 2020).
Machine learning and big data analytics underlie the functioning of AI. The essential condition for optimizing business processes in the company is the choice and algorithms for performing artificial intelligence procedures for implementing business goals: forecasting, segmentation, and natural language processing. Researchers emphasize the role of developing practical AI applications that accelerate business operations, increase productivity, enhance marketing activities, and increase sales. Analyzing the mood of consumers and their emotions through natural language processing (NLP) helps managers get to know their customers better and improve the experience of their interaction with the brand, which ultimately strengthens the company's market position and competitiveness. By introducing AI into their business processes, companies also discover many potential prospects and new competitive advantages in the market (Akerkar, 2019).
2.2. Artificial social intelligence and public opinion mining
At the end of the 20th century, scientists paid attention to forming an essential direction of company management—artificial social intelligence. Artificial intelligence and human intelligence could have social action, social structure, and sociality thanks to the cognitive abilities of their agents. The system of artificial social intelligence defines interference and dependence, as well as types of coordination: reactive, anticipatory, one-sided, two-sided, selfish and collaborative. The development of artificial intelligence, the widespread use of machine learning has led to the embedding of AI in products and services around consumers. This raises the need for ethical research on the social side of the mutual interaction of general social and machine intelligence. First of all, this is the concept of “e-trust” in human-AI interactions as an example of relevance for business organizations (Ferrario et al., 2020).
Information about consumer opinion for marketing is a priority in forming knowledge about the client and constructing successful business strategies. The era of big data, with massive databases generated by people daily and in any form, digital tools for extracting valuable business information from them and practical algorithms, opens up unlimited opportunities for companies to extract data on consumer opinions in real-time (UPLAB, 2022).
Modern marketing is already unthinkable without analyzing public opinion in the Internet space, in particular social networks as platforms for various kinds of communication between people, because of which social capital, trust, in particular, trust in leadership opinions is formed. Facebook, Instagram, Google, Twitter, and other digital media giants own their own public opinion analysis tools based on publicly available software libraries and tools such as World2Vec (Doc2Vec), short text, which focus on thematic modeling and extract low-level functions based on deep machine learning approaches (Tsapatsoulis and Djouvas, 2019). Marketers and web analysts move from analyzing the sentiments of so-called tweets and automatically collecting and testing algorithms in real situations in the Twitter API to even more realistic analysis of opinions—on Facebook and Instagram platforms, which are much more popular today among ordinary people. Analyzing tweets and comments of users of social networks to commercial products is an important marketing tool (Tsapatsoulis and Djouvas, 2019).
Processing vast amounts of data, in particular, consumer opinions about products, is beyond human intelligence's control. AI-based marketing platforms like Google and Amazon can process and extract the information necessary to improve business efficiency. Such systems with artificial intelligence can store information about many people and machines from different sources and reproduce it asynchronously and simultaneously (Verma et al., 2021). Moreover, AI can compensate for the failures of current marketing activities and the external pressure of informatisation. Scientists point out that the efficiency of business processes and the accuracy of calculations and forecasts increases, the management decision-making process bears fewer losses, relations with consumers improve, sales grow, and risks and costs reduce due to AI technologies (Chen et al., 2021).
2.3. Social networks as base for marketing emotional AI research
Social networks are a favorable environment for emotional AI. As a phenomenon of the information society of the XXI century, they are nothing more than a social system with many participants and relationships. Social networks are, in fact, market platforms where effective communications are built between all stakeholders of companies: manufacturers, suppliers, and consumers. Social networks are a network format of peculiar services. Based on this format, users build their profiles according to a social platform's rules, choose a circle of other users and share certain information with them, linking the totality of their social contacts with other network users. Social media has revolutionized interactions between people, providing opportunities for meeting social needs in new ways. At the same time, their impact on wellbeing is obviously ambiguous: it depends on how and who uses them (Kross et al., 2021).
Back in the early 20th century, with the advent of radio and television networks, American engineer D. Sarnov formulated a law in which the value of broadcast networks was directly proportional to the number of listeners and viewers (Currier, 2019). Social networks allow people from all occupations to express openly their emotions and feelings in connection with certain situations, personal circumstances, and attitudes to purchased goods and services. Existing research in this area primarily focuses on the controlled analysis of moods and detecting emotions and their modeling from unmarked and unstructured dialogues in social networks. Modern authors propose a comprehensive framework based on the principles of self-structuring artificial intelligence for modeling and analyzing emotions. This structure systematically integrates modeling capabilities at a detailed level with unstructured, un-labeled data from social networks (Adikari et al., 2021).
Modern digital technologies can identify and interpret human emotions by tracking facial expressions, body language and speech. Emotional artificial intelligence becomes a routine part of everyday life, is interwoven into the activities of commercial and government organizations (Monteith et al., 2022). The problem of recognizing human emotions, which means predicting their tastes, preferences, recommendations and behavior through AI, has become one of the most popular in research today. People use various indirect and non-verbal means to convey their emotions. Emotion detection is carried out using facial recognition, physiological signals, variations of speech signals and text semantics in standard databases: JAFFE, CK+, Berlin Emotional Data Base, SAVEE, as well as separately functioning databases (Saxena et al., 2020).
Much information about the dynamics of emotions of people generating messages, reviews and comments is contained in the so-called time series of tonal assessments that arise in the sentimental analysis of the flow of text messages in social networks. The intelligent analysis of such time series allows for finding some patterns of the dynamics of emotions in the answers of customers of companies. Based on the classification method, analyzing the tonality of short time series of tonal assessments reveals situations when customer reviews have positive or negative dynamics. This method by marketers, web analysts and chatbots will significantly increase the level of customer service, and the quality of the product offered (Sydorenko et al., 2020).
2.4. Sarcasm and hidden emotions detection
The most basic hidden emotion in exchanging information between users about a product or service is sarcasm, expressed in words, facial expressions, and voice intonation. Sarcastic comments are transmitted daily through tweets, comment sections on social networks and headlines of news feeds (Sura et al., 2022). The detection of sarcasm in the text is very problematic due to the lack of intonation and voice vibrations. In the digital age, this task is shifted to tokenization and coding strategies used as printed copies to erase objects created by machines. Tokenization is the replacement of a confidential data element with a non-confidential equivalent (token) without independent meaning for use. Companies use pre-processing strategies to detect and correctly interpret emotions: tokenization, evaluation preparation, analytical criticism, and checking records before deleting highlights (Sura et al., 2022).
Subjectively sarcastic offers can carry emotionally acerbic information about products and services. Identifying the types of sarcastic tweets is very valuable in detecting users' moods. Machine learning and deep learning make it possible to identify sarcastic tweets. Recent research has focused on studying various methods for feature extraction and classification of the machine and deep learning, using estimated indicators of accuracy, precision, completeness, and f-score (Kumar and Goel, 2020).
The advantages of automatic identification of sarcasm in collecting and analyzing opinions are causing research enthusiasm today. When sarcasm is automatically identified, the program tries to determine the type of text: sarcastic or neutral (Gupta et al., 2020). The authors conduct the study in two stages. In the first stage, they extract functions related to tonality and punctuation and then use the chi-square test to compile a list of the most valuable positions. In the second stage, the researchers extracted and combined the top 200 tf-idf functions with tonality and punctuation-related functions to search for sarcastic content in a tweet. Scientists have found that if maximum accuracy is achieved using a machine algorithm of support vectors in the first approach, and then maximum accuracy is achieved through the voting classifier in the second approach (Gupta et al., 2020).
Effective analysis of public opinion about products and services through AI has become the basis of marketing strategies today. Researchers, using a scientometric approach, thematic and patent search methods, and extended citation, collect a lot of bibliographic records of opinion mining research, in-depth semantic analysis identify intellectual landscapes and recent events, record citation trends at the level of each domain, assignment of thematic categories, keyword matching, a network of joint citation of documents, and essential articles (Li et al., 2020; Jang et al., 2022). The study of algorithmic and linguistic aspects of collecting opinions is of the most significant interest to the Internet community in terms of understanding, quantifying and applying the emotional orientation of texts. Based on the analysis of recent thematic trends, the authors concluded that the practical application of intelligent opinion analysis: forecasting market value and analyzing product reviews attracts the most significant attention of the community (Tsapatsoulis and Djouvas, 2019; Li et al., 2020; Jang et al., 2022).
The modern literature today presents an extensive review of research in the field of microblogging of social networks with applications to situational awareness, based on which approaches have been developed in such thematic areas as crime, natural disasters, finance, the physical environment, politics, health, and population (Lamsal et al., 2022).
Indeed, with the rapid development of the Internet and in the context of COVID-19 pandemic, microblogs, and micro-influencer marketing as a structure for generating web content are becoming one of the most popular social networks (Gerlich, 2022). A person can simultaneously act as both a producer and a consumer of web content. Many companies betting on customer relationships are increasingly paying attention to the Twitter microblog. Blog marketing has become a separate area of Internet marketing. Microblogging marketing strategies are built based on discussions and the exchange of opinions (Du, 2020).
Relying on the delegation theory, modern authors expand marketing research by studying technological, consumer, and country differences in the formation of demand for consumer goods with AI support. The demand for products generated by artificial intelligence is determined today by utilitarian (related to the effective achievement of a goal), hedonistic (related to emotions) and symbolic (related to consumer self-awareness) value factors (Frank et al., 2021). At the same time, the essential factor in forming consumer demand in the network is the opinion of influential people, the so-called influencers created with the help of artificial intelligence. To compare the effects of consumers' response to influencers using AI and traditional influencers, the authors explore the role of an influencer agency (independent or externally controlled) to test the boundaries of results and determine interactive effects between the type of influencer and an influential agency. Consumers are equally willing to follow influencers or people exerting influence in the real space, and they perceive the level of personalization of any influencer as the same. Moreover, influencers can have a more significant impact on consumers who need unique products (Sands et al., 2022).
Despite the substantial scientific and empirical base of developments in and a wide range of research approaches, the impact of AI technologies—in the social interaction of users of networks and websites—on the profitability of marketing activities should be qualitatively deepened. A methodology for analyzing the influence of public opinion, in particular, micro and nano influencers, on marketing effectiveness should be developed. Modern research contains a gap in the form of a significant underestimation of the possibilities of using AI tools for social interaction through social networks. The contribution of this study to the proposal of a new more effective model for evaluating the use of such tools and to change the focus of marketing toward influencers. The fundamental hypothesis of the study boils down to the following assumption: the phenomenon of social communications and the power of public opinion is that the factor of the number of influencers (in particular, micro and nano influencers, closer and friendlier with their audience) in a company has a much more significant impact on marketing profits than the factor of the effectiveness of the entire marketing system.
3. Materials and methods
3.1. Theoretical background
Figure 1 shows the methodological design of the study.
Consumers' motivation grows daily to be unique and own a unique and original product. In this regard, the creation of narrow niches prevails, including the appearance of opinion leaders (influencers) influencing their audience largely than traditional advertising. Social networks are the activity areas of opinion leaders and the most popular and easily accessible marketing data sources. Social networks are effective platforms for exchanging opinions, recommendations, experience, and knowledge.
The growth of social media platforms provides an inexhaustible and infinitely information-rich source of public knowledge about the world around us, and the issue of processing and analyzing unstructured data, going beyond personal observations and experience into the field of the big picture and situational awareness is fundamental.
Artificial intelligence and machine learning have now become the leading technologies for responding to demand, dynamic pricing, planning and control of product sales. In the future, AI can radically change marketing strategies and customer behavior (Davenport et al., 2020).
Using AI, intelligent data analysis has become a tool platform, which allows managers to extract essential information for studying sales markets, making forecasts of demand and revenue, strategies for optimizing business processes, identifying important cells in them and infusing financial, personnel, and logistical resources into them, reducing time costs. Through AI and the implementation of a unique tonality analysis function, it has become possible to perform a high-speed and practical analysis of public opinion and better understand the mood of customers, their expectations, the degree of satisfaction with the product and the recommendations they leave in the open Internet space.
Natural language processing (NLP) tools allow companies to obtain incomparably more detailed information about the opinions associated with the words in the text and the emotions of consumers hidden in it.
With the help of software tools and a set of data processing methods: artificial neural systems, decision trees, tracking behavioral patterns (in particular, associative rules), anomalies in consumer behavior, consumer classification, associations in product selection, clustering, regression, and forecasting—the impact of data mining on the company's business efficiency is growing.
In the modern technological world, artificial intelligence raises the quality and speed of finding, extracting, processing and managing essential data for the company to an unprecedented high level. Determining customer turnover, identifying consumer opinions and negative online reviews, automating the addition of product tags (tags) to tickets (internal requests) of employees, visualizing the results of data mining by building charts and graphs, etc.—all this is within the power of artificial intelligence as the main engine of modern marketing.
Companies optimize a consumer's path from the genesis of interest in the product to making an actual purchase, repeating it and turning it into a loyal buyer through machine learning and neural networks. According to Salesforce, the number of companies using artificial intelligence in worldwide marketing grew from 29 to 84% in 2018–2020 (Sales Force, 2022).
The investment scale of introducing artificial intelligence in marketing is also striking. Thus, global costs for AI hardware, software and services will exceed $340 billion by the end of this year, and in 2024 will amount to $500 billion (Social Media Strategies Summit, 2022).
The authors should note that justifying such investments is that artificial intelligence can generate and promote high-quality personalized content on any scale. Unlike traditional software, which only automates tasks, AI is capable of self-learning and self-improvement, which reveals the prospects for enhanced growth in the efficiency of business processes.
Artificial intelligence provides companies with such marketing information management technologies as PPC advertising, personalization, predictive analytics and deep learning (Figure 2).
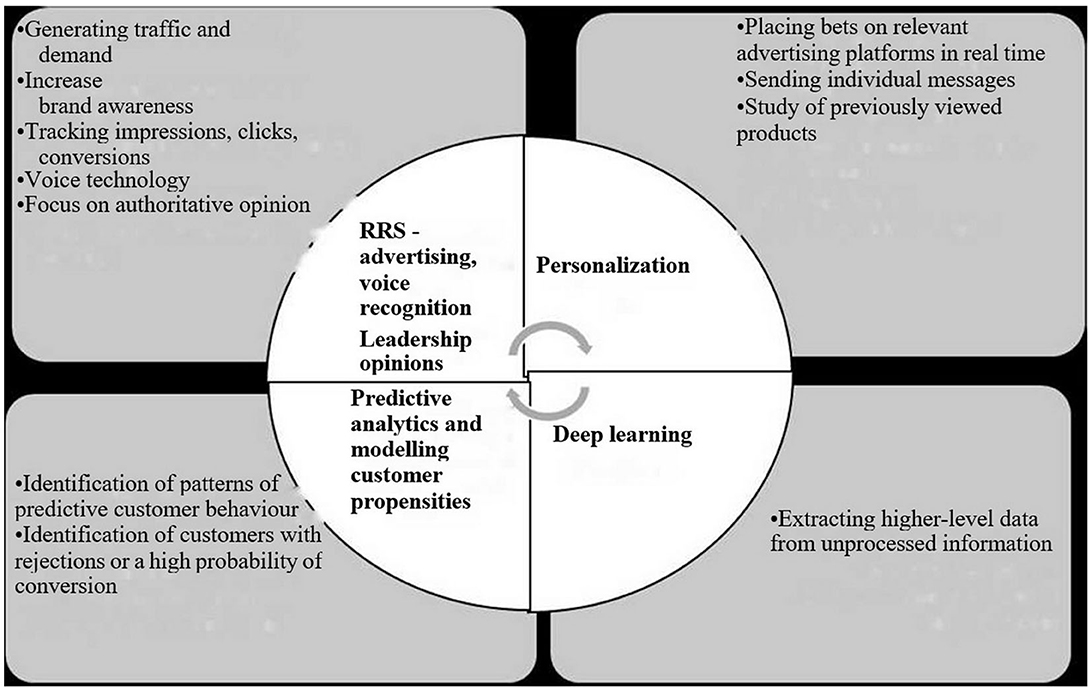
Figure 2. Digital technologies and their role in AI-based marketing information management. Source: developed by the authors.
The process of marketing as a targeted focus on profit growth through AI naturally begins with the stage of reaching the user audience. The current needs of users and an artificial outside intention generate demand to buy a product. Programming media auctions as an automatic purchase of advertising platforms by a company is a very convenient marketing tool that saves time and makes the location process more open.
Based on the knowledge of the target audience, targeted advertising allows for attracting the attention of users. Making clicks by users expands the limits of their knowledge about products and services, increasing the likelihood of purchase. By generating traffic and contextual advertising, companies increase brand awareness and monitor impressions, conversions and user search stories about the product.
Voice technologies as an alternative to visiting a website today increase the effectiveness of companies' relationships with customers through interactive interaction. Every day more and more marketers integrate the functionality of voice recognition technologies into their marketing strategies and invest vast amounts of money in voice marketing. At the same time, more and more users are using voice technologies when making informed purchase decisions, comparative price analysis, and directly adding a product to the cart (Outcode, 2022).
At the end of the coverage stage, when potential buyers get into the so-called sales funnel, content—the site pages that contribute to better user perception of a product or service—becomes the primary way of communicating with it. The conversion level depends on the effectiveness of content management, its timely updating following changes in the product and user sentiment (turning potential buyers into real ones).
In parallel with content marketing, an effective tool for generating demand and subsequent sales is such a flexible promotion tool as influence-marketing, and at all stages of profit growth from marketing activities. Influencers hold the attention of the user audience to a product or service throughout its life cycle. An essential condition for the success of influence marketing is the coincidence of the opinion leader's values with the brand's products he promotes. The advantage of influence marketing is that it embeds advertising into content in a latent form without annoying users' attention and bothering them (Table 1).
Influencer marketing, content marketing, RSS advertising, and voice technologies identify the maximum number of user interaction points with a brand and its products and contribute to lead generation—converting ordinary site visitors into leads (potential buyers).
Marketers use AI to model the propensity to buy at the action stage when a user is involved in the sales funnel and makes the first purchase. Modeling is combined with predictive analytics and aggregation of large amounts of data. It may include the construction of predisposition models: the predicted duration of the customer's “life”, the expected share of the wallet, the propensity to engage, the tendency to unsubscribe, the propensity to convert, and the propensity to become a loyal customer.
When artificial intelligence has extracted and processed user data, targeted advertising is activated, working with vast amounts of data and classifying users according to their expectations, emotions, and moods. As a result, an advertisement is generated that considers all the information about the buyers.
At this stage, potential customers are evaluated and leads are counted. AI applications are involved in the process, for example, Lead Gen. They serve as effective tools for capturing leads based on answers provided by site visitors to qualified questions about needs, plans, budget and the ideal product (Figure 3).
At the conversion stage (the appearance of repeat buyers), companies must be as maneuverable as possible in the changing external environment to retain old and attract new customers. Marketers use a dynamic pricing strategy as a flexible approach to revaluing the cost of products in response to market conditions. In addition, at this stage, it is practical to re-target users who have visited the site and have not made a purchase. Re-targeting is a flexible marketing mechanism for reminding about products that previously interested users. Web personalization creates a unique individual experience of user interaction with a brand and helps companies to identify the qualities of users that are important for building strategies (intentions, geolocation, behavioral patterns, and personal data) and, accordingly, offer them the most adaptive relevant value proposition to their needs. Chatbots are effective for product promotion at this stage. They act as “virtual interlocutors” with programmed answers, working on social networks (Instagram, Facebook), messengers (Viber, Telegram, WhatsApp) and platforms for conferences (Skype, Zoom).
At the stage of attraction, companies have a certain number of loyal customers, and some buyers change their status, ceasing to purchase products. Therefore, companies provide predictive customer services when they use knowledge from the action stage, based on predictive Big Data analytics (predictive analytics), and launch those products and services that customers are more likely to buy. At this stage, managers use specialized applications and technical solutions to accelerate and automate marketing processes for more effective brand promotion: they send content e-mails and work with social networks.
Thus, the opinion of influencers is the only tool that permeates AI-based marketing at all stages of consumer interaction based on the authors' scheme. Micro and nano influencers are the cheapest and, therefore, more cost-effective. They have a close friendly audience of subscribers and established close trust relationships with them. As a rule, the conversion rate (conversion of subscribers into genuine buyers) is relatively high due to the advertising activities of micro and nano influencers. Therefore, small companies, having market flexibility, often resort to financing this marketing expense item.
Based on AI, a fundamentally different, more productive and flexible marketing information system (New Marketing Information System, NMIS) is being created. The basis of its functioning is the social capital of the virtual space: social relations of trust and exchange of marketing information through leadership opinions and comments on goods and services. On the one hand, it helps to form new demand segments and increase sales. On the other hand, information about user opinion serves as an ideal tool for technological and innovative improvement of the product offer, ultimately leading to sales growth.
Information filters are the most critical factor limiting the exchange of opinions about products and services between users and the receipt of marketing information to company managers.
In the marketing information system, barriers often arise that distort and restrict marketing information—the so-called information filters: physical, semantic, and pragmatic. Their tracking and accounting in analyzing marketing information are essential. Physical filters filter out information through the senses to the extent of who a person is: audile, visual, or kinaesthetic. The semantic filter filters out some of the information to the extent of a person's ignorance of certain words, terms or concepts. Finally, the pragmatic filter selects only the information that is useful and necessary for a person.
ASI is based on AI capabilities often exceeding human intelligence abilities, social capital as a relationship of trust of social network users to the authoritative, experienced opinion of public opinion leaders—influencers, around whom a loyal consumer audience centers.
Artificial intelligence shortens the socio-psychological distance between people, contributing to the accumulation of consumers loyal to Φ brand. The old traditional ways of advertising have become obsolete in the digital age. Effectiveness of marketing information dissemination, product advertising, leadership opinions about its quality.
The use of marketing information should generate and strengthen its competitive advantages for a company: in pricing, in possession of unique knowledge and technologies, in its scientific and applied research, intangible assets (patents, licenses, and software), corporate reputation, and consumer confidence in a brand. In addition, marketing information should be the basis for strategic decisions made by management and forecasts effectively processed by artificial intelligence.
Companies allocate considerable funds for implementing the tools and technologies of marketing strategies described above, expecting to compensate for these expenses with profit growth. Maximizing profits from marketing activities and the growth of its index is a confirmation of the effectiveness of marketing strategies and the correctness of the choice of a complex of artificial intelligence technologies.
3.2. Study design
The design of the study is aimed at determining the degree of influence of public opinion formed by social networks through influencers on the effectiveness of marketing strategies of companies from various fields of economic activity when implementing AI tools for advertising. Artificial intelligence relies on public opinion research and the influence of influencers to create advertising actions. The main task was to separate the influence of public opinion from the impact on the growth of the company's revenues of other factors from the field of marketing activities. To do this, we used a survey of heads of marketing departments of large companies implementing AI for advertising, as well as a weighted average assessment of marketing strategies by external experts. The estimates obtained in this way during the survey were compared with statistical data on company income indices and correlations were sought between individual factors and the number of influencers. A number of special tools were used to make the research methodology as objective and representative as possible.
3.3. Sampling
From 02.08.2022 to 01.10.2022, the authors drew sample 1. It included five managers of Internet marketing services of five Russian companies in popular market niches: fashion, beauty, fast food, travel, and electronics. Sample 2 consisted of 12 external experts. Experts were selected based on the experience of marketing research in international markets for more than 10 years; the presence of at least 5 publications in peer-reviewed academic journals over the past 5 years; experience in the implementation of AI tools in marketing for at least 3 years from the current time and the presence of successful implemented marketing cases or analytical studies that have served to make successful corporate decisions over the past 5 years. All external independent experts selected according to these principles gave their personal consent to participate in the study. They studied the features of Internet marketing of five companies actively implementing AI in advertising, sales and social information activities: a (fashion), b (beauty), c (travel), d (fast food), and e (electronics).
The 5 companies that participated in the study were selected by the 12 above mentioned experts based on a consistent Delphi dialogue during 3 consecutive discussion sessions until a consensus list was reached. A prerequisite for the selection was that none of the experts either at the time of the study or in the past had ties or common interests with the companies selected for the study. The managers of the companies participating in the study also provided consent to participate in the study.
3.4. Survey
The researchers asked employees of marketing and sales services of five companies actively implementing AI in business processes to fill out a questionnaire with the stages of consumer interaction over time and the AI technologies and tools used by the companies. Five heads of Internet marketing departments attended the survey. They had complete information about introducing AI technologies in product promotion (one manager from each company). The evaluation of each tool's implementation indicated the frequency and popularity of its use on a scale from 0 to 3.
3.5. Statistical processing
The companies provided data on the dynamics of marketing profits for 2015–2020, expressed in indices (Figure 4) and used to analyze their correlation with the quantitative factor of public opinion in social networks.
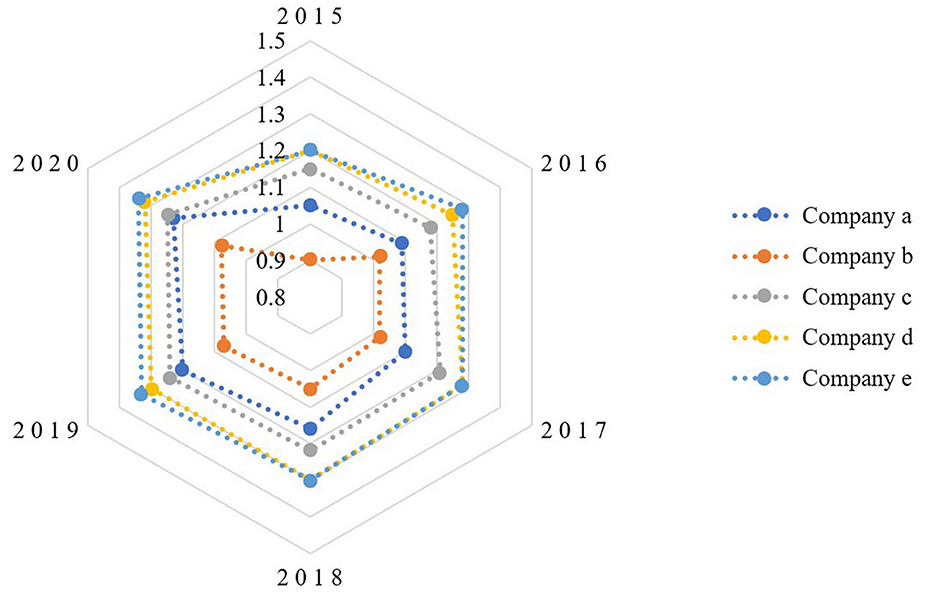
Figure 4. Index of profit from marketing activities of the companies implementing AI in 2015–2020. Source: developed by the authors.
Marketing profit MPc is the target function of maximization and the difference between gross income Ic in the current period and costs Cc for marketing activities in the current period:
The marketing profit index IMP is calculated as the ratio of marketing profit MPc in the current period to marketing profit MPb: in the base period
By analyzing the elements and directions of the new NMIS marketing system, the authors developed AI indicators (sub-indexes) of marketing research as key competitive advantages of the companies. They derived an integral NMIS performance indicator based on them (Table 2).
The rating scale is from 0 to 5 points. Suppose all the evaluation indicators given in the tables are qualitative parameters and are amenable to expert evaluation on a scale. In that case, the micro and nano influencers' factor is quantitative and analyzed separately.
The NMIS effectiveness was evaluated based on weighted average expert assessments of the marketing system's performance indicators. External experts were invited to study the marketing plans and strategies of companies a, b, c, d and e over the past 3 years, based on which they derived estimates of the effectiveness of using the competitive advantages of companies in marketing areas such as collection and analysis of marketing information, its diffusion, digital technologies of its management. Number of experts = 12. The authors compiled a table of weighted average expert estimates of the effectiveness of the NMIS indicators.
K1 = 1/n Σ(x1,..., x6), where n = 6
K2 = 1/n Σ(x7,..., x14), where n = 8
K3 = 1/n Σ(x15,..., x18), where n = 4
n is the number of subindexes.
Thus, the integral efficiency indicator was derived as the arithmetic mean of its three components: complex indicators of the effectiveness of marketing information analysis, its diffusion and digital management.
3.6. Limitations
The study's limitations reside in focusing on subjective expert assessments of the effectiveness of introducing AI technologies in marketing. In addition, this study of AI technologies does not consider how an analytical tool such as deep ultra-precise neural networks influences selling. The companies surveyed do not currently use this tool.
3.7. Ethical issues
Confidentiality of information is observed in the study. The companies' names that participated in the survey and their financial information are confidential (the researchers analyze their dynamics of marketing profits).
4. Results
Table 3 contains the survey results.
Based on a survey of marketers, the most popular and frequently implemented AI tool is social interaction and social network leaders' opinion in generating demand, and the appearance of first, repeat and loyal customers.
The list of implemented AI tool presented in Table 3 was obtained by listing the managers of the participants and then discussing between them the content of each specific tool in the framework of defining common terminology and understanding of each of the tools. This discussion was also based on the Delphi methodology until there was a general consensus on the understanding of each tool and agreement on determining whether the tool corresponding to the common understanding was actually successfully implemented by a particular company. The assessment of the quality of the impact of each AI tool by each of the managers is his subjective assessment, based on practical experience.
Table 4 presents the results of the expert assessment of the NMIS effectiveness.
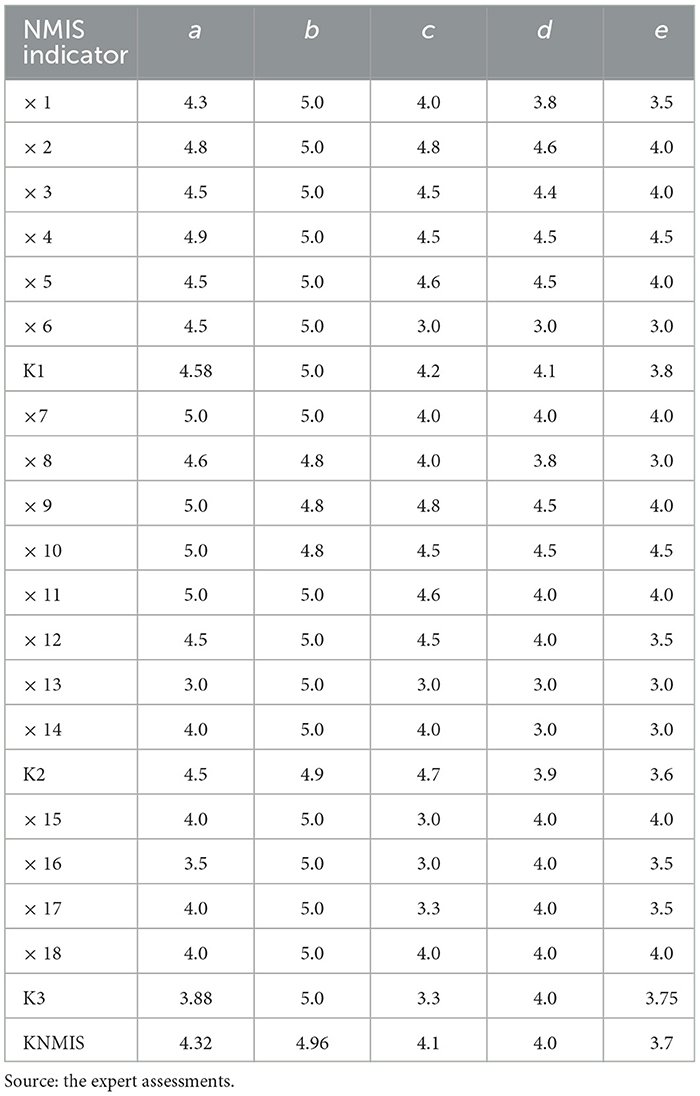
Table 4. Weighted average expert estimates of the effectiveness of the marketing system of companies implementing AI in marketing, 2020.
The assessment was also obtained through a comprehensive discussion by the experts of the specific data and actions of each individual company and the results of the implementation of the relevant tools until the experts reached a consensus on the assessment.
Table 5 contains the NMIS performance factors.
The table does not contain a consensus estimate, but the weighted average estimates of external experts in relation to each of the parameters. Parameter designs for this table can be viewed in Table 2 (rightmost column).
Table 6 presents qualitative factors of marketing profit, aggregated into the integral KNMIS indicator and the quantitative factor of public opinion (the number of micro and nano influencers).
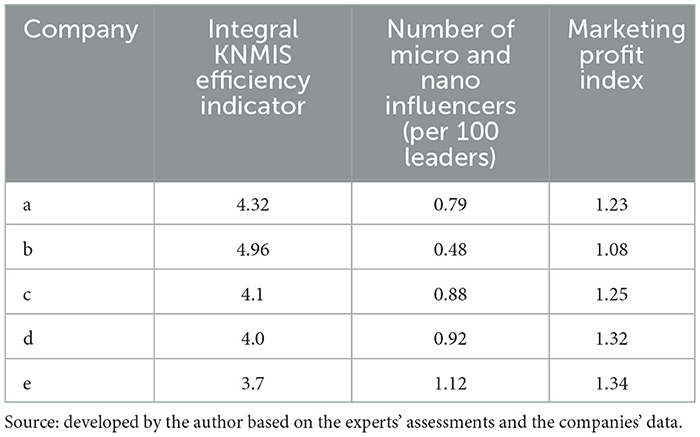
Table 6. Profit factors from marketing activities of the five companies using AI technologies in 2020.
As can be seen from the diagram in Figure 5, the higher a company's profit index, the greater the number of micro and nano influencers, while the complex factor of the effectiveness of the entire marketing system does not demonstrate a direct impact on profit growth. Thus, the fundamental hypothesis of the study is confirmed.
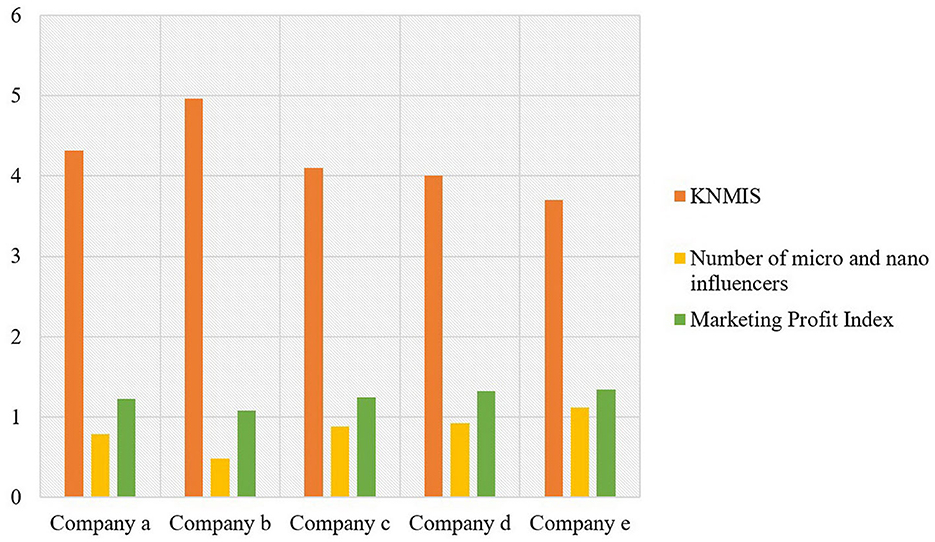
Figure 5. Comparison of the integral indicator of the effectiveness of the marketing system, the marketing profit index and the number of micro and nano influencers. Source: developed by the authors.
The revealed correlation coefficients between the marketing profit index and the number of micro and nano influencers in companies over the past 5 years have confirmed a strong direct link between the factor of public opinion in social networks and profit growth (Table 7).
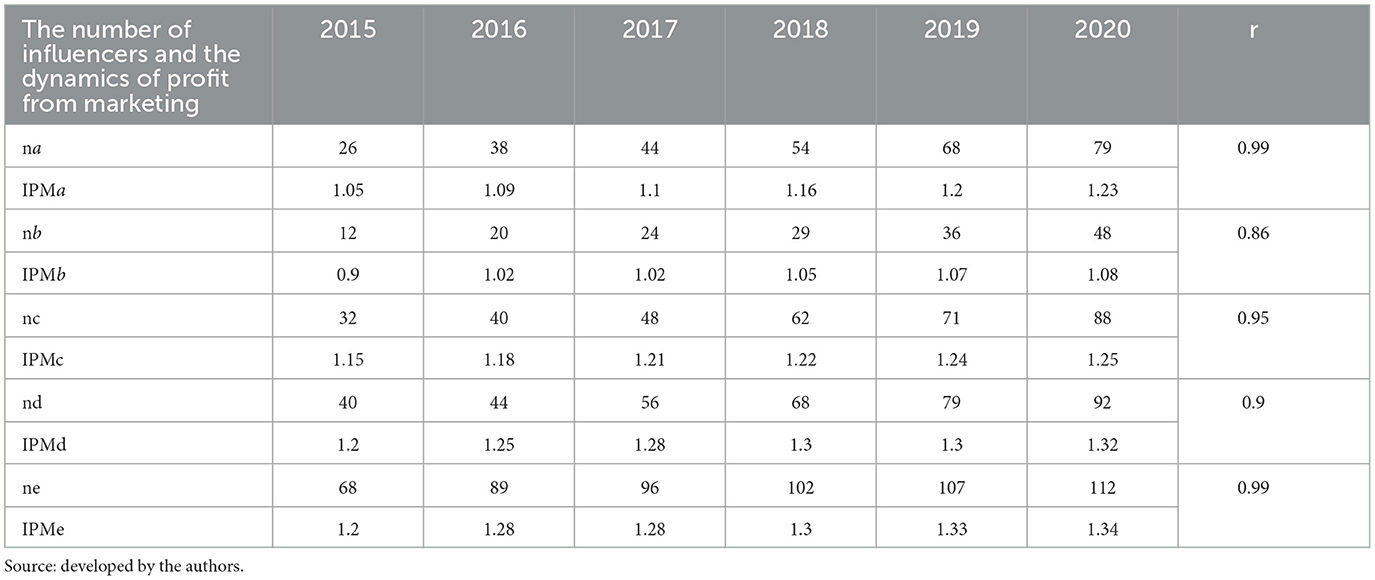
Table 7. The influence of the number of micro and nano influencers (na, nb, nc, nd, ne) on the dynamics of the marketing activity profit index (IPMa-IPMe) of the five Russian companies (a-e) using AI and social networks in their product promotion, 2015–2020 (correlation coefficients, r).
5. Discussion
This research rests on the thesis that the use of artificial intelligence technologies increases the productivity of business processes, in particular, the effectiveness of marketing activities, which is also noted in the publications of many other authors (Chen et al., 2021).
This study examines the influence of AI on the effectiveness of marketing activities in the context of the stages and directions of its implementation. This approach correlates with much modern research. The authors study marketing, starting with the generation of ideas and the development of innovations (Jarek and Mazurek, 2019), from the perspective of strategic planning, technological modernization and customer focus (Gil et al., 2020). This approach proceeds from the thesis that AI represents the most important competitive advantage in the struggle of brands for leadership positions in the market (Akerkar, 2019).
Analyzing the effectiveness of modern AI marketing, we take into account the factor of machine deep learning and intelligent analysis of public opinion, which is consonant with the work of researches, who studied this factor from the perspective of structure, methods, including those based on neural networks (Dargan et al., 2020).
The most popular social media factor (Facebook, Instagram, Twitter) is today a strategic marketing tool (Tsapatsoulis and Djouvas, 2019), while artificial intelligence is the only means of high-speed and efficient processing of huge amounts of consumer opinion data (Verma et al., 2021). Data on social interaction, experience and knowledge through social networks are essential for analyzing marketing information and constructing marketing strategies and customers' wellbeing (Kross et al., 2021).
At the same time, blog marketing and trends in microblogs and micro-influencers change consumer opinion about products (Du, 2020). They are an essential component of Internet marketing today. The leadership opinions in social networks and users' response to marketing information generated and disseminated by influencers affect the success of the marketing activities (Sands et al., 2022).
Through AI and social platforms, user emotions are modeled and conveyed, which affects public opinion about goods and services and generates demand and sales (Adikari et al., 2021). Analysis of public opinion through artificial intelligence improves the quality of customer service and helps to improve the consumer properties of goods promoted on the market (Sydorenko et al., 2020).
Intelligent machine analysis of public opinion about the quality of goods and services through the fixation of citation events at the level of each domain contributes to the construction of market price forecasts by marketers, a more detailed study of opinions about products in long period observation (Li et al., 2020).
6. Conclusion
New digital options increase the productivity of marketing activities: from extensive market research to personalized communications with consumers and website users. Neural networks accelerate and increase the efficiency of market research processes, optimize media plans, improve the quality of monitoring of target groups of consumers and increase the impact of communications with key stakeholders: consumers, suppliers, partners and employees. Artificial intelligence elevates the usual marketing functions—analytical, production, logistics, and sales—to a higher level of implementation and profitability.
Artificial intelligence forms a qualitatively new, more effective marketing information system (New Marketing Information System, NMIS) based on Artificial Social Intelligence (ASI). ASI is based on the interaction of human and artificial intelligence. With the interaction of wise management and technical and software analysis of marketing information that meets current progress, the NMIS confers the advantages of technologies for effective product promotion, expansion of new market niches and strengthening analytical, production, logistics, and marketing functions.
Based on weighted average expert assessments of the introduction of AI tools for product promotion and information provided by marketers of the five companies operating on artificial intelligence platforms, the authors confirmed the study's hypothesis about the strong influence of the number of micro and nano influencers. Even in those companies where the integral efficiency indicator was higher and the number of influencers was lower, the marketing profit index turned out to be lower, and the profit index turned out to be higher in those with a minor integral efficiency indicator with a large number of influencers. Thus, the main factor of profit from marketing activities turned out to be the factor of public opinion, expressed in the number of micro and nano influencers closest to their audience, and not an integral indicator of the effectiveness of the entire marketing system, including sub-indices of qualitative marketing parameters, such as qualified managers, software, and systematization of information, the level of development of the bank of models and market forecasting, the availability of a single information database, the level of compliance with trade secrets, PPC advertising, personalization, predictive analytics, and so on.
AI tools provide opportunities for more precise and targeted influence through influencers. On the other hand, they create challenges related to the fact that the influencers themselves can, for various reasons, lose the trust of the audience and the factors influencing this need to be predicted. Further research can be aimed at studying the dynamics of the number of influencers and factor analysis of individual significant factors linking their number and other indicators with the success of marketing strategies and mediatize influence of AI.
Data availability statement
The original contributions presented in the study are included in the article/supplementary material, further inquiries can be directed to the corresponding author.
Ethics statement
Ethical review and approval was not required for the study involving human participants in accordance with the local legislation and institutional requirements. Written informed consent to participate in this study was not required from the participants in accordance with the national legislation and the institutional requirements.
Author contributions
MG: methodology, supervision, writing—original draft preparation, and validation. WE: formal analysis, resources, visualization, and validation. KS: conceptualization, data curation, writing—review and editing, and validation. All authors contributed to the article and approved the submitted version.
Conflict of interest
The authors declare that the research was conducted in the absence of any commercial or financial relationships that could be construed as a potential conflict of interest.
Publisher's note
All claims expressed in this article are solely those of the authors and do not necessarily represent those of their affiliated organizations, or those of the publisher, the editors and the reviewers. Any product that may be evaluated in this article, or claim that may be made by its manufacturer, is not guaranteed or endorsed by the publisher.
References
Adikari, A., Gamage, G., De Silva, D., Mills, N., Wong, S. M. J., and Alahakoon, D. (2021). A self structuring artificial intelligence framework for deep emotions modeling and analysis on the social web. Future Gener. Comput. Syst. 116, 302–315. doi: 10.1016/j.future.2020.10.028
Akerkar, R. (2019). Artificial Intelligence for Business. New York: Springer. doi: 10.1007/978-3-319-97436-1
Chen, L., Jiang, M., Jia, F., and Liu, G. (2021). Artificial intelligence adoption in business-to-business marketing: toward a conceptual framework. J. Bus. Ind. Mark. 37, 1025–1044. doi: 10.1108/JBIM-09-2020-0448
Currier, J. (2019). The network effects Bible (Updated 2022). NFX. Available online at: https://www.nfx.com/post/network-effects-bible (accessed October 15, 2022).
Dargan, S., Kumar, M., Ayyagari, M. R., and Kumar, G. (2020). A survey of deep learning and its applications: a new paradigm to machine learning. Arch. Comput. Methods Eng. 27, 1071–1092. doi: 10.1007/s11831-019-09344-w
Davenport, T., Guha, A., Grewal, D., and Bressgott, T. (2020). How artificial intelligence will change the future of marketing. J. Acad. Mark. Sci. 48, 24–42. doi: 10.1007/s11747-019-00696-0
Du, J. (2020). An analysis of the value and strategies of microblog marketing. J. Finance Res. 4, 102. doi: 10.26549/jfr.v4i1.3513
Ferrario, A., Loi, M., and Vigan,ò, E. (2020). In AI we trust incrementally: A multi-layer model of trust to analyze human-artificial intelligence interactions. Philos. Technol. 33, 523–539. doi: 10.1007/s13347-019-00378-3
Frank, B., Herbas-Torrico, B., and Schvaneveldt, S. J. (2021). The AI-extended consumer: technology, consumer, country differences in the formation of demand for AI-empowered consumer products. Technol. Forecast. Soc. Change 172, 121018. doi: 10.1016/j.techfore.2021.121018
Gerlich, M. (2021). COVID-19 induced changes in consumer behavior. Open J. Bus. Manag. 9, 2425–2451. doi: 10.4236/ojbm.2021.95131
Gerlich, M. (2022). Micro-influencer marketing during the COVID-19 pandemic: New vistas or the end of an era? J. Digit. Soc. Media Mark. 9, 337–353.
Gil, D., Hobson, S., Mojsilovi,ć, A., Puri, R., and Smith, J. R. (2020). “AI for management: An overview,” in The future of management in an AI world (Cham: Palgrave Macmillan) 3–19. doi: 10.1007/978-3-030-20680-2_1
Gupta, R., Kumar, J., and Agrawal, H. (2020). “A statistical approach for sarcasm detection using Twitter data,” in 2020 4th international conference on intelligent computing and control systems (ICICCS) (IEEE) 633–638. doi: 10.1109/ICICCS48265.2020.9120917
Jang, H., Park, S., and Yoon, B. (2022). Exploring technology opportunities based on user needs: Application of opinion mining and SAO analysis. Eng. Manag. J. in press. doi: 10.1080/10429247.2022.2050130
Jarek, K., and Mazurek, G. (2019). Marketing and artificial intelligence. Centr. Eur. Bus. Rev. 8, 46–56. doi: 10.18267/j.cebr.213
Kross, E., Verduyn, P., Sheppes, G., Costello, C. K., Jonides, J., and Ybarra, O. (2021). Social media and well-being: Pitfalls, progress, and next steps. Trends Cogn. Sci. 25, 55–66. doi: 10.1016/j.tics.2020.10.005
Kumar, Y., and Goel, N. (2020). AI-Based learning techniques for sarcasm detection of social media tweets: State-of-the-art survey. SN Comput. Sci. 1, 318. doi: 10.1007/s42979-020-00336-3
Lamsal, R., Harwood, A., and Read, M. R. (2022). Socially enhanced situation awareness from microblogs using artificial intelligence: A survey. ACM Comput. Surv. 55, 1–38. doi: 10.1145/3524498
Li, X., Wang, B., Li, L., Gao, Z., Liu, Q., Xu, H., and Fang, L. (2020). Deep2s: improving aspect extraction in opinion mining with deep semantic representation. IEEE Access 8, 104026–104038. doi: 10.1109/ACCESS.2020.2999673
Monteith, S., Glenn, T., Geddes, J., Whybrow, P. C., and Bauer, M. (2022). Commercial use of emotion artificial intelligence (AI): Implications for psychiatry. Curr. Psychiatry Rep. 24, 203–211. doi: 10.1007/s11920-022-01330-7
Outcode (2022). How will voice recognition technologies affect the development of marketing in the future? Available online at: https://outcode.ru/blog/kak-tekhnologii-raspoznavaniya-golosa-povliyayut-na-razvitie-marketinga-v-buduschem (accessed October 15, 2022).
Retailers (2022). Influencer marketing spending hits $3,69 billion in 2021. Available online at: https://retailers.ua/news/menedjment/12323-marketingovyie-zatratyi-na-inflyuenserov-v-2021-godu-dostignut-369-mlrd (accessed October 15, 2022).
Sales Force (2022). Turn data into magical, real-time customer experiences with Salesforce Genie. Available online at: https://www.salesforce.com/de/?ir=1 (accessed October 15, 2022).
Sands, S., Campbell, C. L., Plangger, K., and Ferraro, C. (2022). Unreal influence: leveraging AI in influencer marketing. Eur. J. Mark. 56, 1721–1747. doi: 10.1108/EJM-12-2019-0949
Saxena, A., Khanna, A., and Gupta, D. (2020). Emotion recognition and detection methods: A comprehensive survey. J. Artif. Intell. Syst. 2, 53–79. doi: 10.33969/AIS.2020.21005
Social Media Strategies Summit (2022). Data has a better idea. Available online at: https://blog.socialmediastrategiessummit.com/10-examples-of-ai-in-marketing/ (accessed October 15, 2022).
Sura, K., Damera, S., and Posonia, A. M. (2022). “Sarcasm detection in news headlines,” in 2022 6th International Conference on Computing Methodologies and Communication (ICCMC) (IEEE) 1467–1473. doi: 10.1109/ICCMC53470.2022.9754165
Sydorenko, V., Kravchenko, S., Rychok, Y., and Zeman, K. (2020). Method of classification of tonal estimations time series in problems of intellectual analysis of text content. Transp. Res. Proc. 44, 102–109. doi: 10.1016/j.trpro.2020.02.015
Tsapatsoulis, N., and Djouvas, C. (2019). Opinion mining from social media short texts: Does collective intelligence beat deep learning? Front. Robot. AI 5, 138. doi: 10.3389/frobt.2018.00138
UPLAB (2022). Big Data technologies: how to use Big Data in marketing. Available online at: https://www.uplab.ru/blog/big-data-technologies (accessed October 15, 2022).
Keywords: artificial intelligence, emotion analysis, intelligent opinion analysis, microblogging, natural language processing, sarcasm detection, sentiment analysis, social data analysis
Citation: Gerlich M, Elsayed W and Sokolovskiy K (2023) Artificial intelligence as toolset for analysis of public opinion and social interaction in marketing: identification of micro and nano influencers. Front. Commun. 8:1075654. doi: 10.3389/fcomm.2023.1075654
Received: 20 October 2022; Accepted: 22 May 2023;
Published: 15 June 2023.
Edited by:
Muhammad Khalilur Rahman, Universiti Malaysia Kelantan, MalaysiaReviewed by:
Joan-Francesc Fondevila-Gascón, Blanquerna Foundation, SpainEduard Vidal Portés, Universitat Ramon Llull, Spain
Copyright © 2023 Gerlich, Elsayed and Sokolovskiy. This is an open-access article distributed under the terms of the Creative Commons Attribution License (CC BY). The use, distribution or reproduction in other forums is permitted, provided the original author(s) and the copyright owner(s) are credited and that the original publication in this journal is cited, in accordance with accepted academic practice. No use, distribution or reproduction is permitted which does not comply with these terms.
*Correspondence: Michael Gerlich, migerlich@yahoo.com