Data Analysis vs. Data Analytics: 5 Key Differences
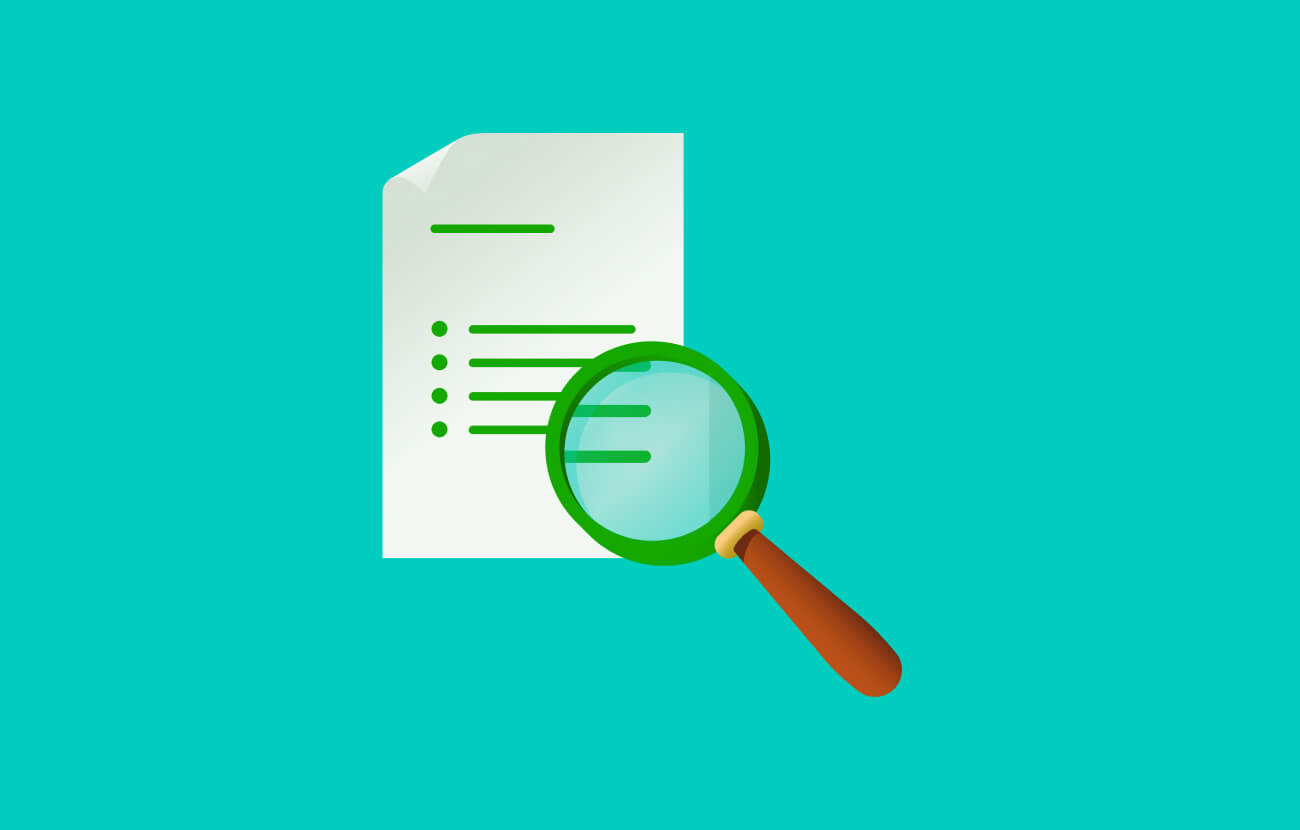
Data intelligence systems are an indispensable asset for modern businesses. Businesses of all sizes—from small enterprises to large multinational corporations—rely on artificial intelligence-based, data-driven insights to make sound business decisions..
However, data science jargon can get pretty confusing at times. Non-technical users who aren’t familiar with computer science might find themselves misusing terms.
Two such terms that continue to stump business owners are data analytics and data analysis. The fact that these terms look and sound so similar makes it easy for non-technical users to confuse the two. However, the difference between them is meaningful. Data analytics and data analysis are two different parts of the data intelligence process, and each has its own methods and objectives.
In this article, we’ll conduct an in-depth examination of these two terms and their unique definitions. We’ll outline the key points of difference between them so that you’re in the know.
What is data analytics?
Data analytics is a broad field in data science in which experts collect data from sources to be standardized, analyzed, and interpreted. Experts use this data to draw actionable conclusions. It represents the entire process organizations undertake, drawing from insights to make better business decisions.
Here’s how the standard data analytics process works:
- A team of key decision makers and data scientists comes together to identify key problems or areas of improvement within a business’s process. For example, data scientists might examine low customer traffic on important holidays like Thanksgiving and Christmas or they might look into an increase in negative customer reviews online.
- Based on these problems, the team decides what data should be collected for study. In our example, the team would recommend both online and offline customer traffic data from the past few years be collected as well as data from online review sites or feedback forms.
- The data is then collected from several sources, such as point of sale (POS) systems, web traffic metrics, online checkout systems, and in-store CCTV cameras. Data should be collected from review sites such as Google Business and Yelp as well as physical feedback forms submitted by customers.
- This dataset contains different types of data from both digital and analog sources. It all needs to be converted into one standard format and checked for wrong or missing values. This process is called the standardization of data.
- Once standardized, specialists pass this data through custom algorithms that pinpoint important values such as most common complaints in negative reviews, products bought most often, and out-of-stock products requested most often near the holidays.
- Based on these results, data scientists draw actionable conclusions. For example, they may recommend stocking up on scented candles and potpourri before the holidays, if those products are likely to run out at that time of year.
Apart from solving specific problems, teams also use regular data analytics to closely monitor important things like team efficiency, net profits, and customer traffic. We’ll explain more about what goes into these actions in the following section.
What is data analysis?
Data analysis is a subset of the broad discipline of data analytics. It’s concerned with the actual treatment of collected data. Data analysis consists of all the processes required to clean and visualize data in order to draw conclusions from it.
Why is data analysis necessary? Raw data, as it’s collected from business systems, may have little value. What data scientists choose to do with the data decides how valuable it will be for the business. Without some treatment, the information contained in raw data is likely to have confusing signals. It can be extremely difficult to record and decipher.
Let’s look at an example.
Say your teams collect data from your POS systems, CCTV cameras, and retail website. You’ll have to use data analysis to transform data from your POS system, which is textual data, into the kind of data your computer can process. Further, the data from your CCTV cameras is purely audio-visual and will need to be processed too. Through data analysis, you’ll carry out standardization processes, which capture the needed data from each file and convert it into one standard format. Once the standardization process is completed, you’re on to the second step. The data needs to be cleaned and modeled correctly.
Data that’s gathered through a storefront must be handled differently than that which is gathered through your website. For a customer, walking into a store and visiting a website are very different experiences, and the data obtained from each destination needs to be treated differently in a data set. Both types of interaction require different amounts of effort on the customer’s part and need to be weighed accordingly while conducting a thorough analysis. Modeling helps you rid your data of these biases in order to facilitate a better, more accurate analysis.
Without the treatment and transformation process, your analysis could present heavily skewed or even entirely incorrect results. That’s why data analysis is such an integral part of the data intelligence processes. Depending on the size and responsibilities of the data analysis team, data analysis may also include interpreting data and suggesting actionable measures to other teams.
Data analysis methods can further be classified into four different categories based on the objective of the analysis. These are as follows:
- Descriptive analysis. This step, also known as data mining, is the most common method of data analysis where large sets of data are captured and analyzed for any patterns that can help scientists gain deeper insight into business processes. This kind of analysis lets specialists find answers to key statistical questions. They might want to know how much revenue the business is generating, how many customers visit the business on average, and how much profit the business is taking away.
- Diagnostic or inferential analysis. As the name suggests, diagnostic inferential analysis determines the root cause of current problems. It involves using data to find out exactly how and why a business process failed.
- Predictive analysis. By utilizing previous data, specialists can use this process to estimate what will likely happen in the future. These predictions are made on the basis of historical data and past consumer trends.
- Prescriptive analysis. This helps specialists gain a statistical perspective on an important business decision. Is it the right time to launch a new product? Prescriptive analysis will answer that question. Can we afford to scale up right now? This type of analysis will help you find out.
5 Key differences between data analytics and data analysis
Data analysis and data analytics pursue different objectives. Each takes a different amount of time to carry out, and they’re used in different circumstances.
While data analytics is a more expansive process that consists of data collection, data validation, and data visualization, data analysis is its subset and is limited to the actual handling and treatment of the data. Here are a few key points of difference between the two processes.
1. Data analysis is a subset of data analytics
For all practical purposes, data analysis is a subset of data analytics. As we said above, data analytics is a broad discipline that includes several processes, such as data capturing (data mining), data analysis, insight generation, and communicating recommendations to the concerned business teams.
Data analytics can be seen as one large business intelligence mechanism in which raw data is turned into helpful recommendations. Data analysis is a small part of this mechanism and is concerned solely with cleaning and analyzing data.
Unlike data analytics, data analysis works within limited boundaries of operation where a specific type of input is needed to generate a specific result (in a standard format). Data analysis can be seen as an integral part of the larger machine, which is data analytics.
2. Data analysis looks at past data while data analytics creates predictive models
Data analysis and data analytics are processes conducted with different sets of objectives in mind. Data analytics is a more open-ended process that aims to ask this question—what will happen next, and how can the business use this knowledge to its benefit?
Data analytics is a predictive process. Specialists gather historical data and study it to find patterns that could generate valuable business insight. It’s up to analytics teams to use their discretion and business acumen to decide what data can be helpful for the organization and how it should be treated. Finding out the answers to these questions helps analytics teams build predictive models that can help make businesses more successful.
Data analysis is a well-defined process that operates between set boundaries. Unlike data analytics teams, analysis teams are given pre-captured and sometimes even lightly processed data to work with. They may also be given information about the operational problems and objectives of the organization. Analysts are then required to use this data to answer the specific questions posed to them. Data analysis, it could be said, is a diagnostic practice in which data is used to find and fix functional faults that are currently happening or have already happened.
3. They are used differently in business
Data analysis and data analytics are used by businesses in separate contexts.
Data analytics leverages all available data sources and uses them to help the business learn from and adapt to consumer demand. It’s a process that’s used to formulate constructive ways in which a company’s operational processes can be optimized to better serve customers and generate more revenue.
On the other hand, the purpose of data analysis is to shed more light on the questions posed by the data analytics team. It’s essentially used to find solutions to problems identified by analytics teams while operating within the data confines set by those teams.
4. Different tools are needed for each
Given the difference in the scopes and processes involved in data analytics and data analysis, it only seems natural that separate sets of business tools would be used to conduct them. Data analytics employs a more comprehensive set of business intelligence tools that helps capture, filter, transform, process, interpret and communicate information.
Here are a few examples of tools used in data analytics:
- R
- Tableau Public
- Python
- SAS
- Apache Spark
- Microsoft Excel
- Google Analytics
- Google Sheets
- ChartExpo
- Microsoft Power BI
- SQL
All-round business analytics tools like Python, Power BI, and Tableau are especially essential to data analytics processes. Learning how to use these tools efficiently to produce actionable insights can prove valuable in your career. You can start by enrolling for certification courses or discovering the programs yourself through how-to guides.
Data analysis tools, on the other hand, are tailored to filter, transform, and process data. These tools are programmed to perform specific analytical operations on large business data sets (also called big data).
Some tools commonly used for data analysis are as follows:
5. The process for data analytics is longer
Data analytics is a longer process with several different steps. A standard data analytics lifecycle includes:
● Data identification
● Data acquisition & filtering
● Data extraction
● Data validation & cleaning
● Data aggregation & representation
● Data analysis
● Data visualization
● Creating data stories
During the course of these steps, business problems are identified and framed as concrete questions, the answers to which are found by capturing and processing gigabytes (sometimes even petabytes) of data.
Data analysis, on the other hand, is a relatively short process compared to data analytics and has three key steps:
● Data cleaning
● Data visualization
● Creating data stories using insights
Data cleaning is an important step of the process. As mentioned, real-world data is of limited value to businesses in its raw form. Raw data is prone to internal biases, discrepancies, and other problems which can hinder effective analysis. Specialists employ several data cleaning techniques to make sure all captured data is standardized and ready for further processing.
Examples of data analytics vs. data analysis
Let’s understand both processes better with the help of a few examples. We’ve categorized the examples according to business industry. We hope you can see the juxtaposition of both processes, exemplified in the same business context.
Healthcare
Data analytics
Let’s say the number of mental healthcare referrals in city X has been increasingly high for the past few years, which is a cause for concern for local authorities.
Analytics teams are hired to find the factors influencing this increase. They’ll use publicly available healthcare and lifestyle data to formulate a strategy. Each municipal block within the city is rated on different parameters like average income, access to quality healthcare, employment and educational opportunities, and overall standard of living.
These parameters are then plotted against the number of mental healthcare referrals in each block. Analysis shows that areas with a lower standard of living and no access to affordable healthcare recorded more referrals. Based on this finding, the analytics team recommends placing a renewed emphasis on providing basic infrastructural and healthcare facilities within these marginalized communities.
Data analysis
When the analytics team tasked with solving the mental health crisis in city X comes up with a concrete plan, it passes the requirements along to the data analysts.
The data analysts collect lifestyle and quality of living data, which is to be filtered and standardized for further analysis. The data is then normalized so that the data analysts can assign specific values to each parameter, and it’s then graphed against the number of mental health referrals. Results are represented by pie charts and histograms that clearly illustrate the correlation between mental health and standard of living.
Finance
Data analytics
Data analytics processes are used in finance to mitigate investment risks and find effective investment strategies that can help investors get the maximum possible returns while staying within their risk parameters.
Let’s look at an example. Venture Capital firm Y is heavily invested in fintech companies, which haven’t been generating expected returns due to an ongoing fintech crisis. Data analytics teams may be hired to help the firm diversify its investment portfolio.
The teams gather data from competing portfolios and global equity markets to identify the sectors that have been doing better. These sectors are then observed in relation to fintech companies in order to pinpoint a relative risk score. For example, traditional banks might be considered high risk since they might lose clients if fintech companies do well. Real estate companies might be considered low risk since they would do well with more amateur investors investing in real estate portfolios using fintech apps. Industries with the lowest risk scores are chosen for investment diversification.
Data analysis
In this scenario, data analysts would be hired to capture and standardize historical revenue and profit data for companies from a specific number of industries. This data would then be measured against the revenue of Y’s fintech companies in order to discover patterns and establish some correlation.
After further investigation, the team would single out industries and companies that generate the best returns during fintech crises. The VC firm would then be advised to invest in these companies to diversify its portfolio going forward.
Which one do you need: data analytics or data analysis?
Both data analytics and data analysis are essential for modern businesses. The two processes shouldn’t be seen as competing alternatives but as different forms of the same data intelligence process.
Data analytics is a good option for businesses that are looking to generate predictive models and need a more holistic data intelligence system that can help them streamline their operations.
Data analysis, on the other hand, is better suited for businesses that already have well-defined problems and are just looking to diagnose them and find solutions with the help of business intelligence systems.
Find professional data analysts on Upwork
If you’re looking for a data science professional to help you with your business intelligence needs, Upwork can help!
Upwork features the world’s largest collection of freelance data analysts and data scientists, highly qualified industry professionals with extensive experience. When you’re browsing through our selection of top data scientists, keep in mind that data scientists should be hired for analysis projects. In contrast, data analysts are the right choice if you have a simple analytics project in mind.
You can discover data engineers in two different ways.
- Look through Project CatalogTM, where listings are classified according to the type of work, with specific predefined projects ready to be contracted.
- Browse Talent MarketplaceTM, where you can review individual profiles and select the professional you’d most like to work with.
Log in now to kickstart your next project.
Upwork is not affiliated with and does not sponsor or endorse any of the tools or services discussed in this article. These tools and services are provided only as potential options, and each reader and company should take the time needed to adequately analyze and determine the tools or services that would best fit their specific needs and situation.
Heading
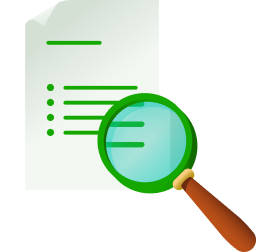
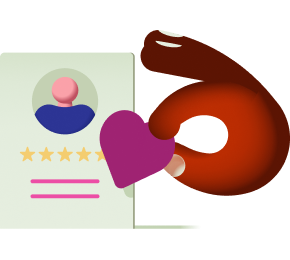
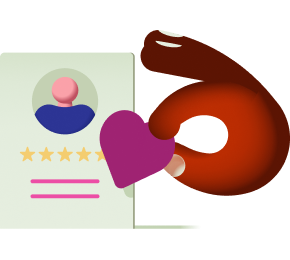
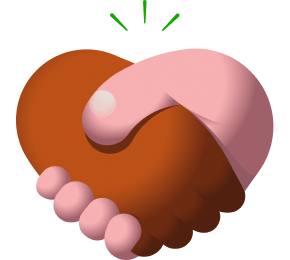

%20(1).png)
Get This Article as a PDF
For easy printing, reading, and sharing.